In an age driven by technological innovation, agriculture faces various challenges, including the issue of wildlife damage to crop fields.
Traditional methods of assessing this damage can be time-consuming and unreliable. To address this issue, a groundbreaking project was initiated by our team of geospatial technology experts. This project aimed to develop machine learning algorithms for wildlife damage assessment, using drone imagery and U-Net model. The project’s significance lies in its ability to automate animal track identification and deliver highly accurate results efficiently. This article explores the relevance and importance of this pioneering project.
The Challenge of Wildlife Damage
Wildlife damage poses a significant threat to agriculture, causing substantial financial losses each year. Farmers often rely on labor-intensive methods such as visual inspections or baited camera traps to identify the culprits responsible for the damage. The need for a more efficient and accurate solution was apparent, leading to the initiation of this innovative project. Drones offer a cost-effective, high-resolution, and rapid method for capturing extensive agricultural landscapes. In this project, drones were deployed to capture imagery of crop fields, providing a comprehensive view of the damage and wildlife activity.
U-Net Model: The Core of Innovation
At the heart of this project is the U-Net model, a convolutional neural network designed for image segmentation. Unlike traditional CNNs, U-Net excels in producing highly precise segmentations with fewer training images. This feature makes it an ideal candidate for identifying animal tracks within crop fields. The innovation enables more accurate detection and classification of damage, setting the stage for proactive wildlife management.The success of this project can be attributed to our dedicated team that worked on developing and fine-tuning the U-Net model. Their efforts have led to a system that differentiates between various animal tracks and accurately assesses the extent of damage within crop fields.One of the key takeaways from this project is the remarkable efficiency it brings to wildlife damage assessment. Automation reduces the time and effort required for data collection and analysis, ultimately enabling a quicker response to mitigate further damage. Farmers can now receive highly accurate reports on the type and extent of wildlife damage within hours, allowing them to take timely action to protect their crops.Moreover, the accuracy achieved by the U-Net model far surpasses what can be achieved through manual inspection or camera traps. This level of precision is invaluable, as it allows for targeted wildlife management strategies that can help deter wildlife from causing further damage and reduce the need for costly, indiscriminate control methods.
The Challenge of Wildlife Damage
Lorem ipsum dolor sit amet, consectetur adipisicing elit, sed do eiusmod tempor incididunt ut labore et dolore magna aliqua. Ut enim ad minim veniam, quis nostrud exercitation ullamco laboris nisi ut aliquip ex ea commodo consequat. Duis aute irure dolor in reprehenderit in voluptate velit esse cillum dolore eu fugiat nulla pariatur. Excepteur sint occaecat cupidatat non proident, sunt in culpa qui officia deserunt mollit anim id est laborum. Sed ut perspiciatis unde omnis iste natus error sit voluptatem accusantium doloremque laudantium.
A Bright Future for Agriculture
The collaboration on this project represents a significant leap forward in the integration of machine learning and geospatial technology in agriculture. It is a testament to the potential of innovative solutions in addressing age-old challenges. The ability to develop a machine learning model to automate animal track identification and deliver highly accurate output efficiently is a game-changer for the industry. This project is not only relevant but also an example of how technology can revolutionize agriculture. It demonstrates how harnessing the power of artificial intelligence and cutting-edge imaging technology can yield insights that were previously unimaginable. As agriculture continues to evolve, it’s projects like these that pave the way for a more sustainable and productive future.
Conclusion
The collaboration to develop machine learning algorithms for wildlife damage assessment, using drone imagery and the U-Net model, represents a turning point in the agricultural industry. The fusion of drone imagery and the U-Net model brings unprecedented efficiency and accuracy to the identification of animal tracks in crop fields. With this innovative approach, farmers now have a powerful tool at their disposal to combat wildlife damage effectively. The project’s success is a testament to the dedication and expertise of our team behind it, and it offers a glimpse into the exciting future of technology in agriculture.
AUAV, a leader in drone technology, specializing in drone manufacturing, services, and training, AUAV empowers industries with cutting-edge solutions.
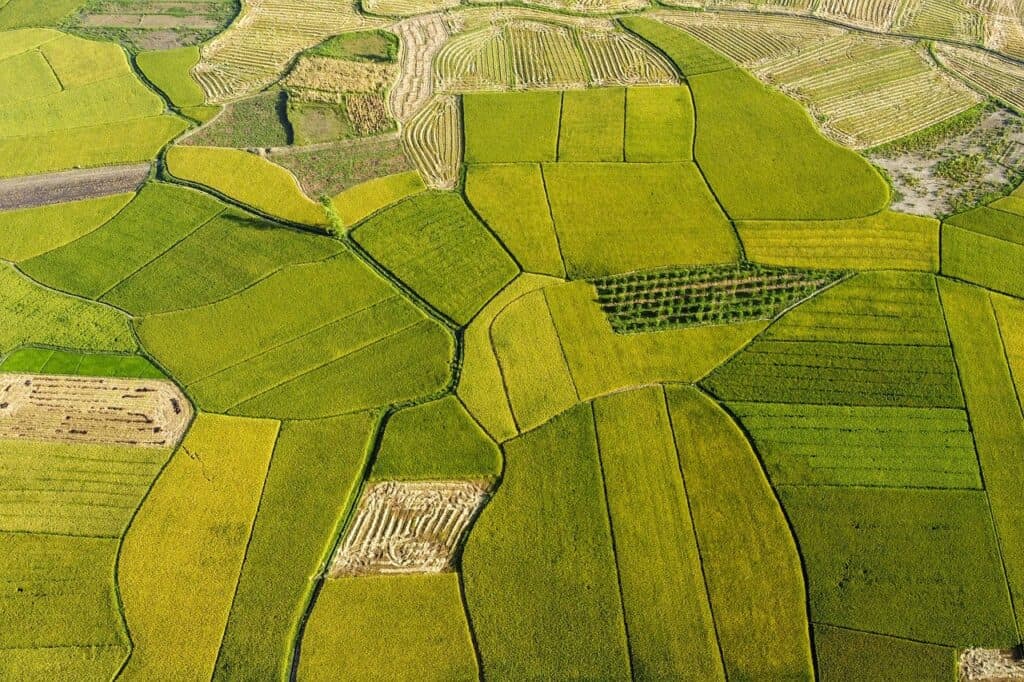